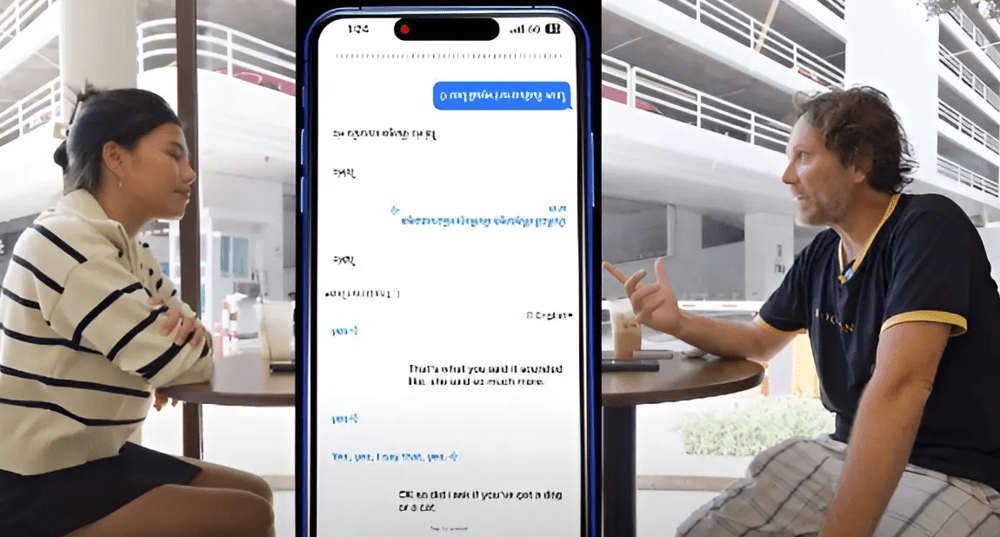
Exploring Translation Technology: Its Evolution from Past, Present, and Future
In the field of language services, the advancement of translation technology marks a significant breakthrough. Starting from simple tools like bilingual dictionaries to the sophisticated AI-driven tools available today.
The progression of translation technology illustrates the overall trends in the language industry and the growing need for translations that are not only fast and precise but also readily accessible. According to a study by Nimdzi Insights, there are currently over 700 language technology solutions available.
Are you interested in how far translation technology has come and what it could achieve in the past, now, and future? This blog post will delve into key technological developments and explore potential future applications for this rapidly evolving field. Be sure to continue reading!
Understanding Translation Technology
Translation technology utilizes sophisticated software and tools to support professional translators and businesses with their localization requirements. Generally, this technology leverages complex algorithms that yield more precise and efficient translations, enhancing overall quality. Such tools range from Translation Management Systems (TMS) and Machine Translation (MT) software to Computer-Assisted Translation (CAT) tools. Machine Translation software, also known as Automated Translation, is a prominent example in the translation field.
It employs neural networks and artificial intelligence to learn from multiple languages, which incrementally increases the accuracy of translations. Recently, this technology has advanced notably in natural language processing, facilitating more effective communication across global populations. Conversely, CAT tools aim to streamline the translation process for professionals. These tools enable translators to conveniently manage translation memories, glossaries, and other essential resources, which helps in reutilizing existing content. Therefore, CAT tools not only save time but also improve the accuracy of translations. The evolution of translation technology has transformed the industry, making translation and localization services more accessible, cost-effective, and precise. This fast-evolving field continues to have a profound impact on global business practices. However, the early days of translation technology were quite different. Let's take a look at the history of translation technology.
From Manual to Machine Translation: A Historical Perspective
The development of translation technology extends back thousands of years. Below is a summary of key milestones in translation technology, starting from manual techniques to the introduction of machine translation:
15th Century: The Beginnings of Manual Translation
The origins of translation technology date back to ancient times when texts were translated by scribes and interpreters across different languages. This method was typically slow, required significant manpower, and was frequently error-prone. Despite these challenges, it established the foundation for more advanced translation tools, most notably with the invention of the printing press.
With the 15th-century invention of the printing press, the translation process was transformed, enabling mass production and distribution of translated texts. This innovation sparked a surge in demand for translation services and led to the rise of professional translators.
The Early Days of Mechanical Translation (1930s)
Long before it became a reality, there was already a dream of machines that could effortlessly translate texts between languages. This vision started becoming a tangible possibility in the 1930s.
In 1933, Georges Artsrouni in France and Petr Troyanskii in Russia, who had no contact with each other, each secured patents for devices resembling early versions of MT. These early machines were quite basic and could only translate words in their simplest forms without adjusting for grammatical changes like declensions and conjugations.
Troyanskii’s invention, for example, required a typist to replicate words in the target language, an operator to mark grammatical roles, and an editor to refine the output into readable target language text. At that time, without the aid of computers, this technology was little more than an enhanced bilingual dictionary.
But the landscape was about to change. By the mid-1940s, pioneers like Warren Weaver envisioned the potential of computers to streamline the translation process. In 1947, Weaver suggested leveraging statistical methods, information theory, and cryptographic strategies from the war to facilitate automated translation using computers.
Introduction to Machine Translation Systems (1950s)
Following that, institutions like the Massachusetts Institute of Technology started to invest in MT, appointing the first dedicated MT researcher in 1951. The peak of these early endeavors was the renowned Georgetown experiment, a groundbreaking public demonstration of MT by computer. The team from Georgetown University, in collaboration with IBM, developed a tool capable of translating Russian into English, albeit with the Russian transliterated into Latin script. They demonstrated this with 60 sentences, which were translated effectively but were not yet practical for regular use. Today, we often encounter grandiose assertions about MT achieving parity with human translation. However, it’s worth remembering that such ambitious projections are not a modern phenomenon. The Georgetown team, for example, optimistically predicted they would perfect their device in just five more years, based only on their initial success with 60 Romanized Russian sentences.
The Early Development of RBMT (1960s – 1980s)
Despite their imperfect technology, researchers at Georgetown and IBM had built considerable momentum in the field of MT. This progress, however, came to a standstill in the United States during the 1960s. The Automatic Language Processing Advisory Committee (ALPAC) issued a report in 1966, which argued that MT was too expensive to warrant additional research because it did not perform as well as human translators. As a result, funding for MT research in the United States was significantly reduced, though developers in other countries continued to pursue their MT projects. During this time, multiple MT approaches were tested, with Rule-Based Machine Translation (RBMT) becoming the favored method. RBMT depended on detailed grammatical and lexical knowledge from each language and used a specific set of rules tailored to each language.
Initial RBMT systems included France's Textile Institute's TITUS and Canada's METEO system. Even though the ALPAC report slowed down research in the U.S., it didn't halt it entirely—SYSTRAN, established in 1968, adopted RBMT and worked with the U.S. Air Force on Russian-English translation projects during the 1970s.
RBMT was the dominant MT approach at that time, but it was limited by its technology. Better than the technology used in the Georgetown experiments, RBMT's development was labor-intensive as developers needed to manually input language rules. This process often led to translations that were either inaccurate or unnatural, particularly with ambiguous or idiomatic input. In their efforts to improve RBMT, some developers explored new avenues—in 1984, Makoto Nagao in Japan introduced example-based MT. Although not broadly adopted today, this approach showcased the creativity of early MT researchers. It also paved the way for others to explore statistical methods, as will be discussed later. These early innovations underscored the necessity for continuous vigilance, scalability, and maintenance in developing MT systems.
The Evolution of Advanced Methods (1990s – 2010s)
In their pursuit to enhance RBMT, researchers introduced a more effective MT technique: Statistical Machine Translation (SMT).
During the 1990s, IBM researchers, reigniting their engagement with MT, unveiled studies on the earliest SMT systems in 1991. SMT differed from RBMT as it did not necessitate the manual input of rules for each language. Instead, it utilized bilingual text databases to discern patterns in languages that could be transformed into statistical data. This analysis of text corpora enabled SMT to pinpoint the most probable translation choices for specific inputs, offering significantly better performance than RBMT and gaining rapid popularity.
As electronic computers became more common in households, MT systems followed. In 1997, SYSTRAN introduced the first web-based MT tool, making MT accessible to everyone, not just specialists and language professionals. Google Translate, launched in 2006, operated on SMT from 2007 until 2016.
Parallel to SMT's progression, the groundwork for Neural Machine Translation (NMT) was being laid. In the year SYSTRAN released its web-based MT tool, Ramon Neco and Mikel Forcada published an inaugural paper on the “encoder-decoder” framework, setting the stage for NMT. By 2003, the University of Montreal's researchers had developed a neural network-based language model, but it wasn't until the advent of the sequence-to-sequence (Seq2Seq) model in 2014 that NMT started rivaling SMT.
Google Translate Introduced NMT (2016)
NMT rapidly advanced to become a refined MT tool, with Google Translate adopting this technology in 2016. NMT systems utilize more extensive corpora than SMT and excel in translating lengthy texts with intricate sentence structures. However, NMT also demands substantial computational resources and time and may falter with content that falls outside its training data.
While NMT marks a significant departure from early experiments like those at Georgetown, it's crucial to value older MT methods. Today, developers still employ SMT to check the applicability of their training data, and RBMT is seldom used on its own for practical applications.
With ongoing developments in MT, integrating various MT techniques has proven advantageous: a hybrid MT strategy might combine RBMT, SMT, and NMT to enhance translation accuracy. This approach is particularly beneficial for languages with scant training data, where RBMT can provide a basic translation that SMT and NMT can subsequently refine. Debugging neural systems proves more challenging than SMT due to the extensive data involved in NMT, which creates opacity issues. An essential takeaway from NMT's early development is the vital need for abundant, high-quality data to build effective MT systems.
In August 2017, DeepL was launched as a convolutional neural network translator. Today, it is globally acknowledged for its high accuracy and supports translations across more than 800 language combinations in 31 languages.
ChatGPT and Beyond (2020s)
In the 2020s, innovations like ChatGPT are emerging as potential game-changers in the field of localization and machine translation (MT). These tools, exemplified by OpenAI's GPT series featuring models like ChatGPT and the recently introduced GPT-4 LLM, are built on extensive neural networks and offer advanced functionalities beyond just translation.
Why is Translation Technology Important in the Business World Today?
Translation technology has reshaped the way businesses function within the modern global economy. The role of precise and effective translation technology is critical, particularly with the expansion of e-commerce and increased worldwide connectivity.
Reliable voice translation devices enable more effective communication with clients, partners, and stakeholders, overcoming language obstacles. A key advantage of translation technology is its capacity to improve communication, empowering businesses to penetrate new markets and broaden their customer base. It automates the translation of languages, allowing for instantaneous communication with new audiences. This technology also supports international collaboration, simplifying the process for businesses to engage with global partners and stakeholders.
Moreover, translation technology plays a vital role in reducing costs and boosting productivity. Businesses can avoid the expense and effort of training bilingual employees or hiring translators by investing in automated translation devices that perform both quickly and accurately. These devices reduce translation expenses and facilitate better communication and more effective dissemination of information. Language translation technology is essential for businesses because it enables global collaboration, diminishes communication barriers, and increases productivity.
Looking Ahead in Translation Technology
The future of translation technology is an engaging subject that has generated a lot of interest within the industry. Undoubtedly, swift progress in Natural Language Processing (NLP), Artificial Intelligence (AI), and Machine Learning (ML) has revolutionized translation. A noteworthy trend in this field is the growing importance of AI-powered translation tools. These tools, powered by deep learning algorithms and neural networks, can now handle the translation of complex content with high levels of accuracy. Yet, enhancing the technology to accurately capture subtleties and translate idiomatic phrases remains an ongoing challenge. Looking ahead, translation technology is poised to incorporate more advanced application programming interfaces (APIs) and cloud-based solutions. Such integrations make it possible for content to be translated smoothly across different platforms and apps. There will likely be a broader embrace of processes that integrate machine-assisted human translation. By employing machine translation for initial content translation, human translators can dedicate themselves to more intricate tasks like editing, proofreading, and ensuring quality, which in turn markedly enhances their productivity and efficiency.
Final Thoughts
In short, translation technology is an incredible tool that is constantly advancing and getting better. It helps people around the world communicate more easily, breaks down language barriers, and broadens cultural understanding. At Digital Trans-Asia, we are ready for the future steps of this journey and are actively enhancing our platform to ensure you have access to the most recent innovations in translation technology.