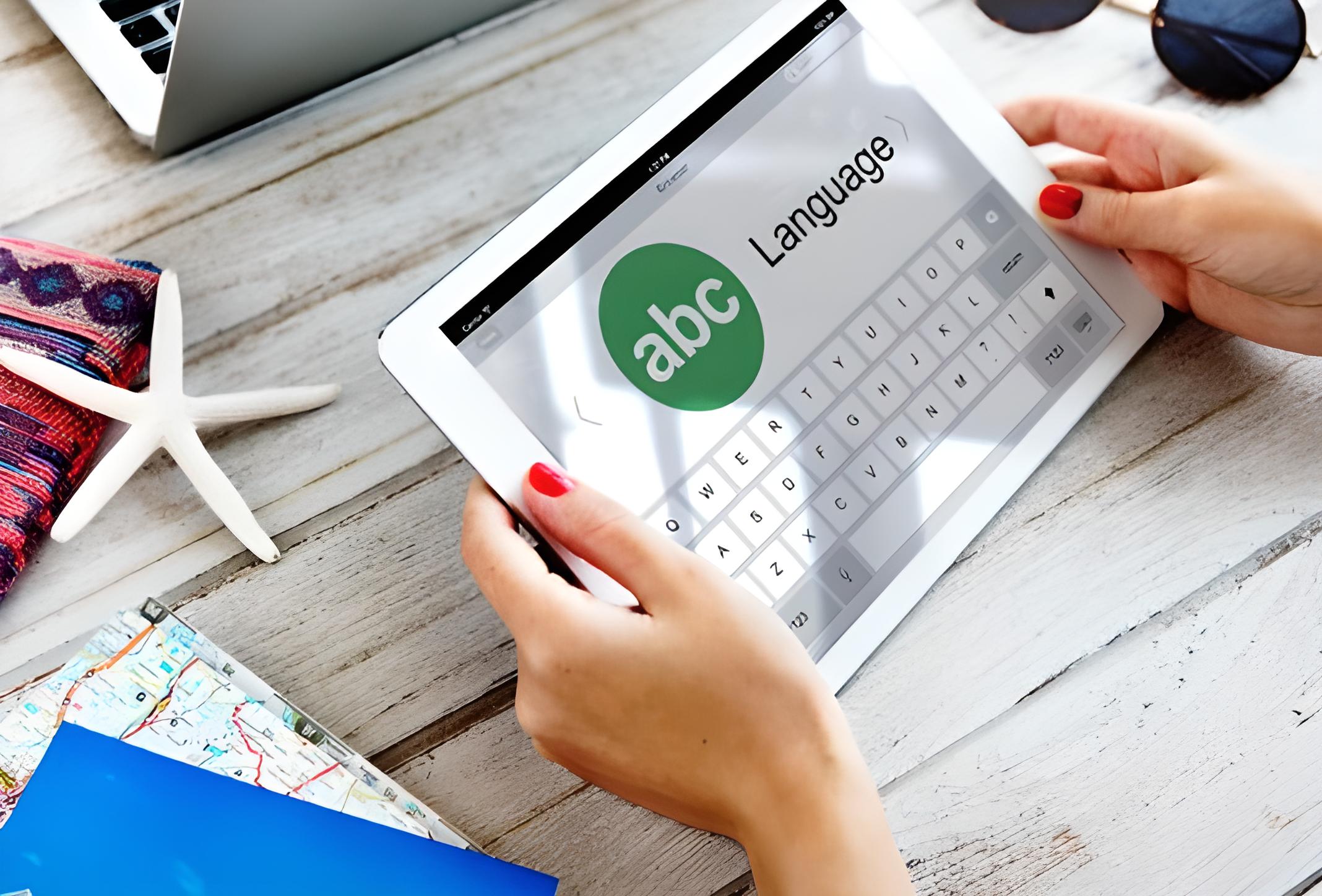
How do the Large Language Models (LLMs) Impact the Translation Industry?
The utilization of Large Language Models (LLMs) and Machine Learning (ML) in the realm of language translation has resulted in notable enhancements in quality, precision, and efficiency. In contrast to older translation systems, these models demonstrate exceptional proficiency in comprehending and producing text that closely resembles human language, thereby yielding more refined and precise translations.
They prove especially adept in languages characterized by intricate patterns, homonyms, compound words, or situations where meaning is heavily influenced by context. These strides are propelling us towards a future where language barriers become obsolete.
Instances of translations from Japanese, Chinese, German, French, and Italian into English exemplify this transformation, showcasing the promising trajectory of translation technology. Within the confines of this article, we aim to facilitate a deeper understanding of the influence of large language models (LLMs) on the field of translation, shedding light on how accurately translation impacts the analysis of ratings and reviews. Keep reading to gain insights!
What are Large Language Models (LLMs)?
A Large Language Model (LLM) is an artificial intelligence system equipped with the ability to comprehend and generate human language by processing vast amounts of text data. Utilizing a transformer model, consisting of neural networks with self-attention capabilities, LLMs can grasp the relationships between words and phrases in the text.
The training process of LLMs involves extensive text data, enabling them to capture complex patterns in language and perform various language-related tasks. With billions of parameters, LLMs can provide consistently relevant responses in context, including language translation, text summarization, answering questions, and even supporting creative writing.
An exemplar widely recognized in the realm of large language models is the GPT (Generative Pre-trained Transformer) series developed by OpenAI, including GPT-3. These models boast billions of parameters, empowering them to comprehend intricate language patterns and connections.
During the training phase, the model is exposed to a varied and extensive array of datasets, facilitating its acquisition of grammar, context, semantics, and even the subtle nuances inherent in language.
In general, Large Language Models (LLMs) represent a potent emerging technology with the capacity to transform various industries. The translation sector is no different, and LLMs are poised to bring about a substantial change in the methods employed for the creation and delivery of translations.
Characteristics and Proficiencies of Large Language Models (LLMs)
Here, we will examine the essential attributes and capabilities that render LLMs formidable instruments within the translation and localization industry:
1. Language Understanding
Large Language Models demonstrates exceptional proficiency in comprehending language, attributed to the sophisticated architecture and extensive training of their deep learning models. Each model undergoes training using vast and extensive datasets, allowing them to grasp the subtleties of grammar, syntax, and semantic relationships. This capability empowers them to accurately interpret and process human language.
2. Language Generation
LLMs possess the ability to produce language responses resembling human expression. When provided with a prompt, they can generate text that is both coherent and contextually fitting, closely resembling the natural flow of human language. This proficiency renders LLMs valuable in applications such as content generation, chatbots, and virtual assistants.
3. Transfer Learning
Next, LLMs utilize the strength of transfer learning, allowing them to apply knowledge acquired during pre-training on extensive datasets. The initial pre-training phase exposes the models to extensive data, facilitating the acquisition of a comprehensive understanding of language. Following this, LLMs can undergo fine-tuning for specific tasks, programming languages, or domains using smaller, task-specific datasets, thereby improving their performance.
4. Multilingual Support
Large language models possess the capability to process and produce text in numerous languages. By undergoing training on an extensive corpus of text data spanning various languages, LLMs acquire the ability to comprehend and generate text within diverse linguistic contexts. This attribute holds significant value in the contemporary globalized landscape, where multilingual communication is widespread.
5. Contextual Understanding
LLMs also possess the ability to comprehend and interpret language within its context. By taking into account the entire input context, these models can discern the relationships between words and phrases. This contextual comprehension empowers LLM to produce responses that are not only coherent and relevant but also contextually fitting.
Illustrations of these features and capabilities can be observed in prominent large language models like GPT-3. With a staggering 175 billion parameters, GPT-3 has demonstrated its proficiency in generating top-tier text, translating languages, responding to inquiries, and even crafting poetry.
How Are Large Language Models Useful for Localization and Translation?
Here are some conceivable impacts of LLMs on the translation and localization industry:
1. Increased Efficiency
Large Language Models have the capacity to automate tasks related to translation and localization, swiftly delivering accurate translations and expediting the process for software UI elements, content, and documentation. Moreover, LLMs contribute to the creation of localized text variations by adapting content to suit the cultural and linguistic nuances of varied target markets.
2. Improved Quality
LLMs demonstrate proficiency in comprehending and generating natural language, a pivotal aspect for managing user input, handling multilingual data, and facilitating language-specific features. These models can also analyze user queries, extract information, and provide responses in the relevant language or dialect.
3. Enhanced the Personalized Experience
LLMs can produce content in multiple languages, encompassing dynamic user notifications, localized marketing materials, or personalized messages. Furthermore, they can tailor content according to user preferences, language settings, or geographic location, thereby elevating the personalized experience for users worldwide.
Read also: What is the Impact of AI in Translation and Localization?
The Challenges of Implementing LLMs for Localization and Translation
Despite the rapid transformation that LLMs have brought to the localization industry, they are not a panacea for automated translation, introducing several challenges to the localization process:
1. Precision & Quality
Although LLMs exhibit impressive language capabilities, they are not flawless and may occasionally generate inaccurate or awkward translations. Upholding high translation accuracy and quality is paramount in the internationalization process, a subset of localization. Therefore, meticulous validation and review are essential to ensure the generated translations are precise, culturally fitting, and contextually appropriate.
2. Domain & Industry Specifics
LLMs like ChatGPT are trained on general datasets sourced from the internet, potentially lacking domain-specific knowledge or terminology. This deficiency can impact the accuracy and suitability of translations, especially in specialized industries or technical content. For localization (L10n) or internationalization (i18n) projects necessitating industry-specific terminology, additional data training or customization of LLMs may be imperative, demanding technical expertise and consideration of privacy concerns related to the utilized datasets.
3. Context & Cultural Awareness
Large Language Models might not entirely comprehend the cultural context or sensitivity inherent in specific language constructs, which could result in inaccuracies or unintentional biases in translations. Such inaccuracies have the potential to cause reputational harm or legal complications.
4. Security
Given that prompts, outputs, and training data may include sensitive information, ensuring the security of LLMs is of utmost importance. There is also the concern of malicious manipulation of data, known as 'poisoning,' which could influence the generated outputs.
Case Studies: Successful LLMs Integration in Translation
In order to demonstrate the tangible effects of incorporating LLMs, we will examine some case studies in the translation industry:
1. Newswire’s GPT-4 Integration
The Canadian company, Newswire, has incorporated GPT-4 into its operational process to translate press releases from English to Quebecois French using machine translation. This action reflects an increasing trust in the capabilities of LLMs and their ability to manage translations that are specialized in nature.
2. Research on MTQE and Document-Level Machine Translation
There has been a notable increase in research within the localization sector concerning Machine Translation Quality Estimation (MTQE) and the utilization of LLMs for document-level machine translation. MTQE plays a pivotal role in guaranteeing that translations align with the desired quality standards, while document-level machine translation strives to tackle context-related challenges and deliver more cohesive translations for longer texts.
3. Tencent’s Role-Playing Prompts
The Chinese technology giant, Tencent, has been exploring methods to improve the quality of machine translation through the use of role-playing prompts. By offering distinct scenarios and contexts for translation assignments, models can produce translations that are not only more accurate but also more relevant.
Final Thoughts
The possibilities offered by large language models in the field of localization are extensive. Encompassing high-quality machine translation that captures both context and nuance, as well as context-aware localization ensuring culturally pertinent adaptations.
The adaptability of these models to regional nuances, their efficiency in generating content, and their ability to uphold consistent terminology all contribute to a more efficient and streamlined localization process.
However, akin to any powerful technology, the utilization of large language models in localization demands careful consideration of ethical and responsible practices. It is imperative to address and mitigate biases, prioritize cultural sensitivity, and maintain human oversight to ensure that the technology serves as an enabler rather than a potential source of unintended consequences. For additional insights into localization and other fascinating aspects of the translation industry, continue to stay tuned to Digital Trans Asia. An exploration into the dynamic realm of localization and translation awaits you.